Model selection#

Underfitting#
the model is too simple
the number of parameters is too low
Overfitting#
the model is too complex
the number of parameters is too large
Train and test#
The common way to reveal overfitting is to use train and test datasets.
training dataset \(\mathcal D_{\mathrm{train}} = (\boldsymbol X_{\mathrm{train}}, \boldsymbol y_{\mathrm{train}})\) is used on learning stage:
test dataset \(\mathcal D_{\mathrm{test}} = (\boldsymbol X_{\mathrm{test}}, \boldsymbol y_{\mathrm{test}})\) used for evlaluation of model’s quality:
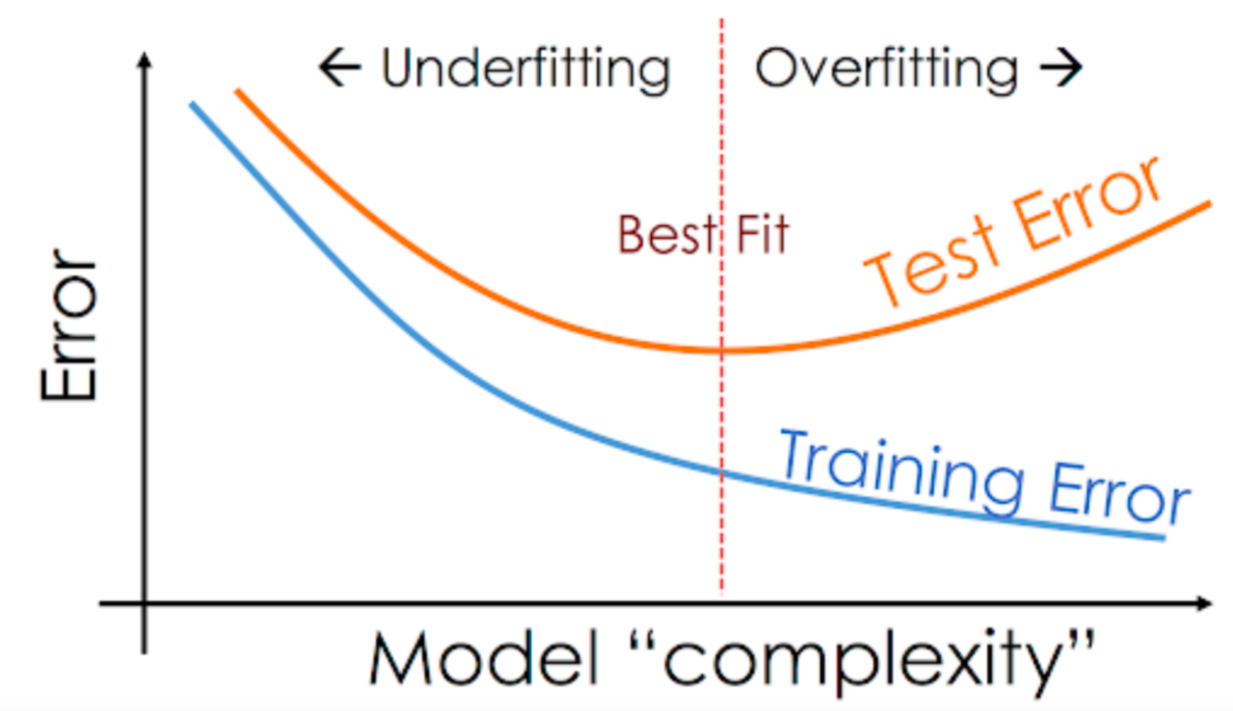
A classical example#
Ground truth: \(y(x) = \frac 1{1 + 25x^2}\), \(-2\leqslant x \leqslant 2\)
Polynomial regression model: \(f_{\boldsymbol \theta}(x) = \sum\limits_{k=0}^n \theta_k x^k\)
Training set: \(X = \Big\{x_i = 4\frac{i-1}{N-1} - 2\Big\}_{i=1}^N\)
Test set: \(\tilde X = \Big\{\tilde x_i = 4\frac{i-0.5}{N-1} - 2\Big\}_{i=1}^{N-1}\)
Loss function — MSE:
\[ \mathcal L_{\mathrm{train}}(\boldsymbol \theta, X) = \frac 1N \sum\limits_{i=1}^N (f_{\boldsymbol \theta}(x_i) - y_i)^2 \to \min\limits_{\boldsymbol \theta} \]What is happening with test loss
\[ \mathcal L_{\mathrm{test}}(\boldsymbol \theta, \tilde X) = \frac 1N \sum\limits_{i=1}^N (f_{\boldsymbol \theta}(\tilde x_i) - \tilde y_i)^2 \]
as \(n\) grows?
The overfitting is a big problem in ML because an overfitted model makes poor predictions. The first signal of the overfitting: \(\mathcal L_{\mathrm{train}} \ll \mathcal L_{\mathrm{test}}\).
Cross validation#
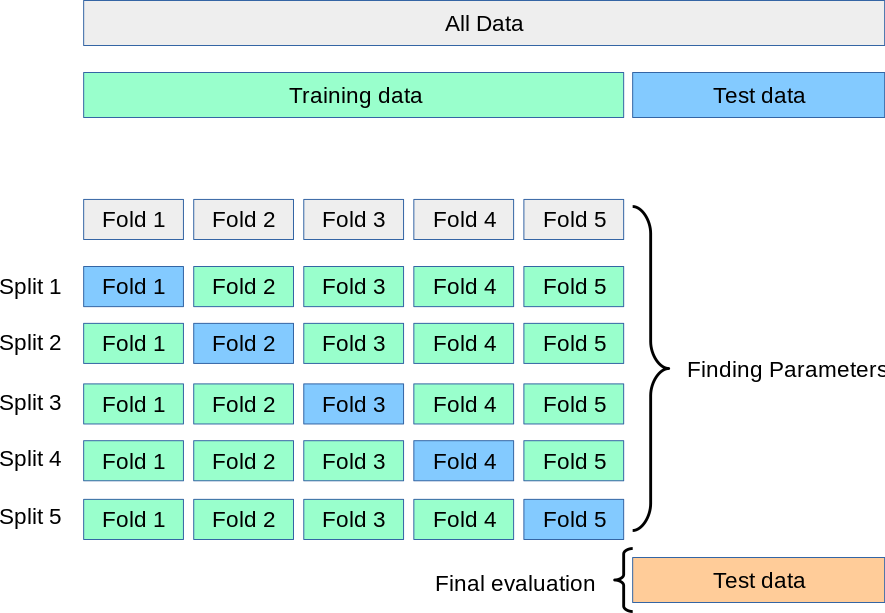